The global demand for web scraping is on the rise. According to Future Market Insights, the market is anticipated to continue growing at a substantial rate, with a CAGR of 15%, over the next decade.
At a time when web scraping is gaining so much traction, wouldn’t it be nice to understand the basics of this process?
Continue reading to learn the basics of web scraping, how it works, and other things you need to know.
What is Web Scraping?
Web scraping is simply the process of automating the extraction of data from the Internet. It involves using software or scripts to visit web pages and gather specific data, such as text, images, or links.
This process, as you can imagine, is crucial for anyone needing comprehensive data for analysis or other purposes.
Don’t want to code? ScrapeHero Cloud is exactly what you need.
With ScrapeHero Cloud, you can download data in just two clicks!
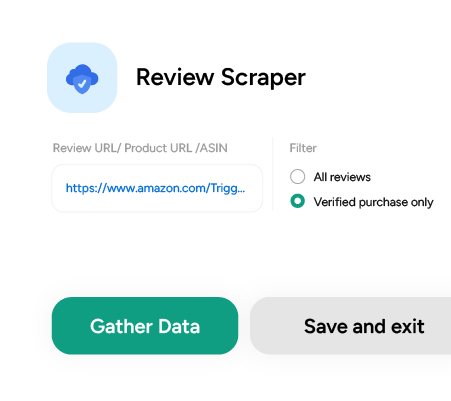
History of Web Scraping
The history of web scraping is closely related to the evolution of the internet and the increasing need to access and process large amounts of web-based data.
During the initial days, manual data collection was common, but later with technological advancements, developers began using scripts to automate simple data extraction tasks from web pages.
The World Wide Web Wanderer, for instance, was one of the first bots to be used on the World Wide Web.
Later, as web pages started becoming more complex and dynamic, more sophisticated tools and software became available.
Web crawlers like Jump Station and AltaVista were developed, and then Google was invented around 1998 and took over as the world’s primary search engine.
The Process Behind Scraping Data from a Website
Now that you know what is web scraping, let us understand what happens when you do web data extraction.
Any web scraping process typically follows these steps:
- Sending a Request: The scraper sends an HTTP request to the target website.
- Receiving the Response: The server responds with the requested HTML page.
- Parsing the Data: The scraper parses the HTML to extract relevant information.
- Storing the Data: Extracted data is stored in a structured format for further use.
Understanding these steps is essential for anyone looking to implement web scraping in their projects.
Key Components of Web Data Extraction
The following are the components that are critical to the process of scraping data from a website:
-
Web Scraper
The tool or script that performs the scraping. Its functionalities include the following:
- Sending Requests: Initiates HTTP requests to target web pages to retrieve HTML content.
- Handling Responses: Receives and processes server responses, managing issues like redirects and errors.
-
Parser
Extracts specific data from the HTML. A parser does the following functions:
- HTML Parsing: Breaks down the HTML document into a tree structure that can be navigated programmatically.
- Data Extraction: Identifies specific elements (e.g., tags, attributes) and retrieves relevant information using selectors or expressions.
-
Data Storage
The systems and formats used to save the extracted data for future access.
- Data Formats: Common formats include CSV for spreadsheets, JSON for structured data, and XML for complex hierarchical data.
- Databases: For larger datasets or more complex queries, databases like SQL (e.g., SQLite, MySQL) or NoSQL (e.g., MongoDB) are used.
-
Scheduler
Automates the scraping process at specified intervals.
- Task Automation: Automatically triggers scraping scripts based on a set schedule (e.g., hourly, daily, weekly).
Types of Web Scraping Techniques
When scraping data from a website, different techniques are employed based on the complexity and requirements of the task.
-
HTML Parsing
HTML parsing involves analyzing the HTML structure of a web page to extract required data.
How It Works: Libraries like BeautifulSoup (Python) are used to parse HTML documents.
Use Case: Ideal for scraping static content from web pages where the data is embedded within HTML tags.
-
DOM Parsing
DOM parsing involves manipulating the Document Object Model (DOM) of a web page to scrape data from a website.
How It Works: To interact with the DOM, you can use tools like Selenium or Puppeteer. These tools can render and manipulate the page as a browser would.
Use Case: This is suitable for scraping data from dynamic web pages where JavaScript is used to modify the DOM after the page has loaded.
-
XPath
XPath is a language used for navigating and extracting data from XML documents. It can also be applied to HTML documents.
How It Works: XPath uses a specific way of writing paths that help you find and navigate elements and their attributes in XML or HTML documents.
Use Case: It is useful for extracting elements that are buried deep within the structure of a web page or document.
-
APIs
APIs provide a structured way to access data directly from a server.
How It Works: APIs offer endpoints that allow to request specific data from a service.
Use Case: This method is preferred when the data provider offers an API.
If you want an API that is specific to your requirements, ScrapeHero can build a custom web scraping API for you.
Programming Languages, Tools, Frameworks, and Services for Web Data Extraction
Various programming languages, tools, and frameworks are available to be utilized when scraping data from a website.
Selecting the most appropriate one based on the specific requirements of your project is important.
Programming Languages for Scraping Data From Websites
Listed below are some of the popular programming languages that are commonly used to scrape data from a website.
Python
Python is one of the most popular programming languages for web data extraction. It is popular for its simplicity, readability, and available libraries and frameworks.
Its libraries include:
- BeautifulSoup
- Selenium
JavaScript
JavaScript is a programming language that can run in browsers. It is suitable for scraping dynamic content rendered by client-side scripts.
The popular libraries offered by JavaScript include:
- Puppeteer
- Cheerio
Java
The programming language Java offers a variety of libraries for web scraping.
Its popular libraries include:
- Jsoup
- HtmlUnit
Web Scraping Tools for Web Data Extraction
Some of the widely utilized web scraping tools used to scrape data from a website are listed below.
ScrapeHero Cloud
ScrapeHero Cloud is an online marketplace by ScrapeHero. It has many easy-to-use web scraping tools and APIs designed to extract data from websites like Amazon, Walmart, Airbnb, etc.
Bonus tip: You can download 25 pages for free using the web scraping tools on ScrapeHero Cloud!
Don’t want to code? ScrapeHero Cloud is exactly what you need.
With ScrapeHero Cloud, you can download data in just two clicks!
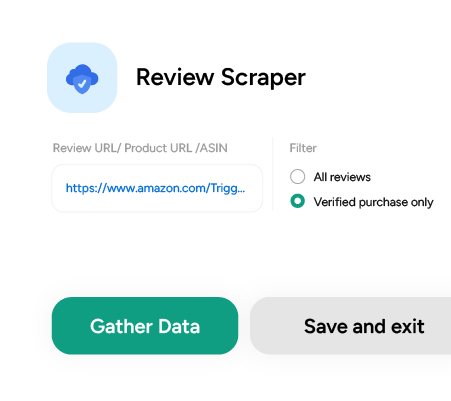
BeautifulSoup
BeautifulSoup is a Python library for parsing HTML and XML documents.
Selenium
Selenium is a browser automation tool that can be used to scrape dynamic content from websites.
Web Scraping Frameworks for Web Data Extraction
A web scraping framework is a platform that provides a foundation for developing software applications. This allows developers to focus on writing application-specific code.
For instance, Puppeteer is a Node library that allows operations without a GUI and hence ideal for web scraping.
Web Scraping Services for Scraping Data From Websites
A web scraping service like ScrapeHero is an expert to whom you can outsource your web scraping requirements.
They take care of all the processes involved in web scraping a website, from handling website changes to figuring out antibot methods to delivering consistent and quality-checked data.
Advantages of Using Web Scraping to Scrape Data From a Website
There are many benefits to using web scraping for scraping data from websites.
- Efficiency: By automating the data extraction process, web scraping helps save time and manual labor.
Web scraping can handle data extraction much faster than humans.
- Consistency: Automated scraping can consistently extract data without the risk of human error, ensuring accuracy and reliability.
- Scalability: Web scraping can handle scraping of large volumes of data across multiple pages and websites.
- Access to real-time data: Web scraping can be set up to collect data at regular intervals. This will provide access to the latest information, such as prices, stock levels, or news updates.
- Cost-effectiveness: Automating data collection reduces the need for manual data extraction, saving on labor costs and freeing up resources for other tasks.
Also, once a web scraping system is set up, the ongoing costs are relatively low compared to manual data collection methods.
Practical Applications of Using Web Scraping
Any business with a digital presence can greatly benefit from web scraping. Below are some of the ways web scraping can be utilized.
Market Research
Companies can use web scraping to monitor competitors’ prices and adjust their own pricing strategies.
By collecting data on product availability, customer reviews, and sales promotions, businesses can identify market trends and consumer preferences.
Academic Research
Researchers can scrape data from websites, online publications, and databases to gather information for academic studies, theses, and research papers.
Researchers can also scrape survey responses, reviews, and other user-generated content to analyze public opinions and behaviors.
Content Aggregation
News websites and applications that require large amounts of data can use web scraping to aggregate news articles from various sources.
Job portals scrape listings from multiple company websites to provide a comprehensive database of job openings.
Sentiment Analysis
Businesses can track mentions of their brand or products across the internet to understand customers’ sentiments towards them.
By scraping reviews and ratings, businesses can gain insights into the level of customer satisfaction.
Challenges in Scraping Data From a Website
When you attempt web scraping, it presents you with certain challenges.
Anti-Scraping Mechanisms
Websites may block IP addresses that make too many requests in a short period, recognizing them as potential bots. This can disrupt scraping efforts.
Many sites use CAPTCHAs to verify that users are human, which can hinder automated scraping processes.
Legal Issues
Scraping data without permission may violate a website’s terms of service or copyright laws.
Laws such as the GDPR (General Data Protection Regulation) in Europe impose strict rules on data collection and privacy, which can impact scraping activities.
Data Quality
Scraped data may have inconsistencies due to varying formats or structures across different web pages.
Some web pages may not load entirely and can lead to scraping incomplete datasets.
Many websites frequently update their layouts and content, which can be difficult to take care of.
Technical Complexity
Writing an efficient and reliable scraping script can be complex, requiring a good understanding of technology and programming skills.
These challenges are bound to happen when attempting to scrape data from a website on your own. It is best to seek the help of a web scraping service like ScrapeHero to get a full-data service.
Legal and Ethical Considerations When Scraping Data From a Website
Web scraping raises several legal and ethical questions.
It’s essential to respect the terms of service of websites and ensure compliance with data protection regulations, such as GDPR and CCPA.
Ethical considerations include respecting the intellectual property rights of content creators and avoiding harm to the websites being scraped.
Best Practices to Follow When Scraping Data From a Website
The best practices when web scraping involves respecting the rights of website owners and maintaining legal compliance.
- Before starting a scraping project, check the robots.txt file of the target website.
- Implement delays between requests to avoid overloading the server.
- Check if the website offers an API and use it to access the required data.
- Use proxy services or rotate proxies to anonymize your requests. This helps distribute the load across multiple IP addresses and can reduce the possibility of being blocked.
Web Scraping and Data Privacy
Data on the Internet could be public, private, or copyrighted. Depending on the type of data you are scraping, scraping could be legal or illegal.
Private or personal data includes any information that can be used to identify a specific individual. Scraping any of this data without explicitly obtaining consent from the individual is considered illegal and violates various data privacy regulations.
Individuals have the right to control their personal information and how it is used, and there are also laws protecting the collection and use of personal information.
Copyrighted data is any information protected by intellectual property rights, specifically copyright law. Even if the content is publicly available on a website, scraping it without permission from the copyright owner is considered a violation of copyright.
Future of Web Scraping
The future of web scraping will greatly be impacted by the advancements in technology, regulatory changes and business needs.
AI and machine learning models might improve the accuracy and efficiency of web scraping by intelligently identifying and extracting relevant data.
Since you have read this article, which explains the A to Z of web scraping data from a website, we assume that this process might be useful to you.
FAQ
Web scraping is the process of automatically extracting or scraping data from websites. It involves using software to gather and parse information from web pages.
Web scraping is legal if done in compliance with a website’s terms of service and applicable laws.
It is important to ensure that the data being collected is not protected by copyright and that privacy laws, such as GDPR, are respected.
Popular web scraping tools used for web data extraction include, ScrapeHero Cloud, BeautifulSoup, Scrapy, Selenium, etc.
To avoid getting blocked, you can follow the following things:
1. Respect the website’s robots.txt file.
2. Throttle your requests by adding delays between them.
3. Rotate IP addresses using proxies.
4. Randomize user-agent strings to mimic human browsing
The ethical considerations to practice when scraping data from websites include:
1. Respecting the website’s terms of service.
2. Avoiding the collection of personally identifiable information without consent.
3. Not overloading websites with excessive requests.
4. Crediting sources when using scraped data.
Python is one of the most popular languages utilized for web scraping due to its simplicity and available libraries.
JavaScript and Java are also commonly used.
Websites can identify scraping activities by monitoring request patterns, user-agent strings, and IP addresses.
Mimicking human browsing behavior while web scraping is often followed to minimize detection.
Turn the Internet into meaningful, structured and usable data
We can help with your data or automation needs